The "Learning" phase in Facebook Ads Manager is the testing phase for delivering your ads to members of your targeted audience. Facebook uses machine learning AI to test and find the most suitable target audience. After going through this phase, the machine learning will be smarter and will deliver effective ads to the right potential customers.
However, many advertisers encounter a common issue: their ad campaigns get stuck in the "Learning" phase. If you’ve ever found yourself asking, "Why is my Facebook ad stuck in learning?" follow this article to help you.
1. Insufficient Conversions
Facebook usually needs around 50 conversion actions within 7 days to exit the learning phase (though this can vary based on your campaign setup and goals). If not enough people take action, your ad will be "stuck" in the learning phase while Facebook waits for more data. There are many reasons why Reasons why you may not be getting enough conversions include:
- Narrow Audience - A very narrow audience limits the algorithm’s ability to find people likely to convert.
- Low Budget - With a budget too low, there may not be enough impressions and conversions for the algorithm to optimize effectively.
- Poor Ad Creative - Ads that fail to resonate with and engage the target audience will lead to poor performance.
- Misaligned Objective - Choosing a goal mismatched to user behavior (e.g. optimizing for purchases when users are not ready to buy yet) will lead to poor performance.
- High Competition or CPM - Expensive ad placements reduce potential reach and conversions, limiting optimization.
To Fix "Learning" limited Ads, make these tweaks:
- Combine multiple ads into just a couple that target bigger pools of people to provide the algorithm with more data to optimize effectively.
- Test out different versions of your ad to see which style attracts more attention!
- Be patient and leave the ad running for longer—as long as it’s getting some attention, not zero.
- Focus your ad on people who recently checked out your website since they’re warmer leads.
- Try setting a lower cost-per-click bid to keep your spending in check.
2. Small Audience Size
It’s likely your ad set took a hit every time you logged in and tinkered with the budget, audience, placement and other settings significantly. With each edit you make, Facebook's algorithm can reset the learning phase on figuring out the best optimization for your campaign. I made this mistake when I first started running Facebook ads. I was so eager to see results that I constantly tweaked the ad sets, desperately trying to speed up the learning phase. But as it turns out, that was having the opposite effect!
It took some trial and error, but here’s what finally did the trick:
- ✔️ Minimized Changes Once Live: I mapped out targeting and creative variations during initial testing. Once the winning combo was live, I made minimal edits over the next 2 weeks.
- ✔️ Edited Strategically Based on Data: With changes limited, I could accurately assess performance metrics day-over-day to guide impactful tweaks.
- ✔️ Consolidated Ad Sets: I combined multiple small ad sets around similar audiences into larger sets so the algorithm could optimize more efficiently.
- ✔️ Increased Budget: I gave Facebook more spend to work with, helping it gather data faster.
3. Low Budget
Facebook needs enough data to determine where and how to deliver your ad effectively towards your goals. This requires not only reach within your audience, but also meaningful engagement and conversions.
If your daily budget only allows for intermittent impressions that seldom convert, the learning phase drags on. The algorithm requires more spending power to test audience segments and placements thoroughly.
Getting My Ad into the “Active” Phase
I implemented these strategies over 2 weeks to help my ad set graduate from learning mode:
- ✔️ Raised Budget to $20/day: The increase allowed wider testing and achieved conversion goals faster.
- ✔️ Consolidated Ad Sets: With fewer variants to test, progress accelerated within the now higher budget.
- ✔️ Expanded Audience: A broader age range and additional interests grew my reachable audience.
- ✔️ Extended Campaign Runtime: Giving it more time at the new budget helped the algorithm optimize fully before I scaled.
With enough data, the algorithm graduated from learning to the “Active” status where I could now scale my Facebook Ads confidently!
4. Complex Campaign Structure
Some common causes of a complex campaign structure that stunts learning are:
- Numerous tightly targeted ad sets fragmented across interests, demographics, behaviors and more
- Testing multiple different combinations of creative, copy, formats, etc.
- Intricate or shifting bidding strategies like aggressive daily budgets or rapid changes in bids
- With so many moving parts for Facebook to analyze, the system simply cannot gather enough signals to progress past the learning period and enter the scaling phase.
To fix this:
- Consolidate ad sets around broader targeting that still reaches your core audience
- Reduce the number of ad variations to identify best performers faster
- Maintain consistent bidding, budget and optimization strategies over longer periods
Essentially, simplify to provide clear signals. This allows the algorithm to hone in on the best options, accelerate learning, and dedicate budget to the highest performing components for greater efficiency and results. Periodically reintroducing complexity allows you to expand reach while preventing ongoing learning issues.
5. Ad Fatigue or Poor Creatives
Ad Fatigue or poor creatives means the same ad is shown too many times to an audience, causing them to skip. This leads to a lack of engagement and prevents Facebook from optimizing for learning and delivery effectively.
To address creative fatigue and quality issues:
- Refresh creatives with new images, videos, copy angles every 2-3 weeks per ad set
- A/B test multiple creatives with different visual styles, captions or video content
- Try an alternate creative concept if current approach sees poor relevance
- Expand target audiences slightly to reach new eyes, take care not to fragment data
- Allocate adequate budget for statistical significance in data
- Closely monitor click-through rate, cost per result, and relevance score
Tackling repetitiveness and improving intrinsic creative quality will re-engage users. Allowing the algorithm sufficient budget exposure to fresh ads helps it identify high-potential options and progress beyond learning into the critical scaling and optimizing delivery phases.
6. Narrow Optimization Goals
Facebook ads often get stuck in a perpetual learning phase when the campaign goals are too niche or specialized. This hampers Facebook's optimization algorithm from gathering sufficient statistically significant data to progress.
Some specific causes include:
- Highly specific target audiences like "left-handed guitarists under 30"
- Optimizing for narrow conversion events like "Add to Cart" clicks
- Focusing spend towards a limited number of goals
With such narrow parameters, the pool of applicable data points remains restricted. Facebook requires wider data sets across broader audiences and conversion actions to identify positive signals and effectively optimize ad delivery.
To push past the learning phase:
- Broaden both audience targeting and placement targeting
- Add higher-funnel events like landing page views as optimization goals
- Increase daily budget to quickly scale data collection
- Use Automatic Placements to give the algorithm full control
Widening your targeting net and the range of measurable events exposes more users to more ad variations at scale. This provides Facebook with enough data depth to determine ideal combinations for pushing users down the funnel.
7. Technical Issues
Getting a Facebook ad out of the learning phase can be frustratingly difficult when technical problems choke optimization behind the scenes. It ends up in a vicious cycle - without enough accurate data at scale for the algorithm to crunch, the ad stays stuck learning basic user patterns.
Typical technical causes include:
- Highly specific small target audiences
- Inadequate daily budget to drive scale
- Fragmented complex campaign setups confusing signals
- Frequent significant adjustments before completion of learning
- Tracking errors from Facebook Pixel or website integration
Without reliable analytics capturing user actions at scale, Facebook struggles to analyze performance signals and graduate ads from learning.
Fixes to overcome data deficiencies include:
- Expand audience targeting beyond very narrow segments
- Increase daily budget to widen reach and strengthen data
- Consolidate fragmented ad sets around unified parameters
- Stabilize elements like creatives, placements and bids for longer periods
- Double check Facebook Pixel and site analytics tracking
Additionally, technical errors preventing accurate analytics can cause data issues. Confirming the Facebook Pixel or site integration correctly records all key conversion events will improve data depth.
Getting stuck in the Facebook Ads learning phase can be incredibly frustrating. If you've tried the tips here around budget, targeting, creatives, consolidation and tracking to no avail, it may be time to bring in an expert. Consider hiring a managed Facebook ads agency like Rent Ads Agency to analyze your account bottlenecks and progress your ads beyond learning into a scaled optimized state.
In conclusion, Getting stuck in the Facebook Ads learning phase can be incredibly frustrating and a waste of your time and money. If you've tried the tips here around budget, targeting, creatives, consolidation and tracking to no avail, it may be time to bring in an expert. Consider renting a Facebook ads agency account like RentAdsAgency.com to analyze your account bottlenecks and progress your ads beyond learning into a scaled optimized state.
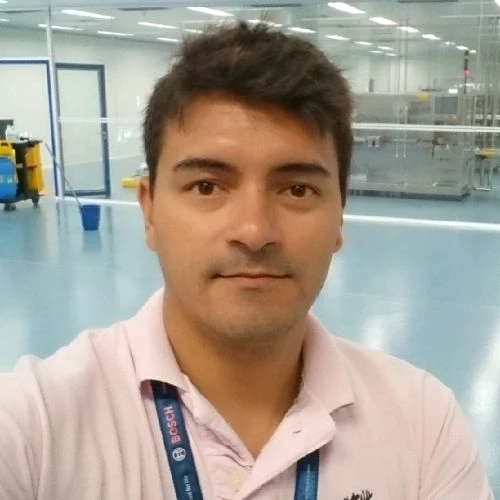
Mohamed Fouad is a full-stack web developer and an entrepreneur who's really into advertising. He is the CEO of Rent Ads Agency, a company that helps businesses reach more customers through advertising. He graduated from Stanford University in 2018 and has over 4 years of experience in the tech industry.